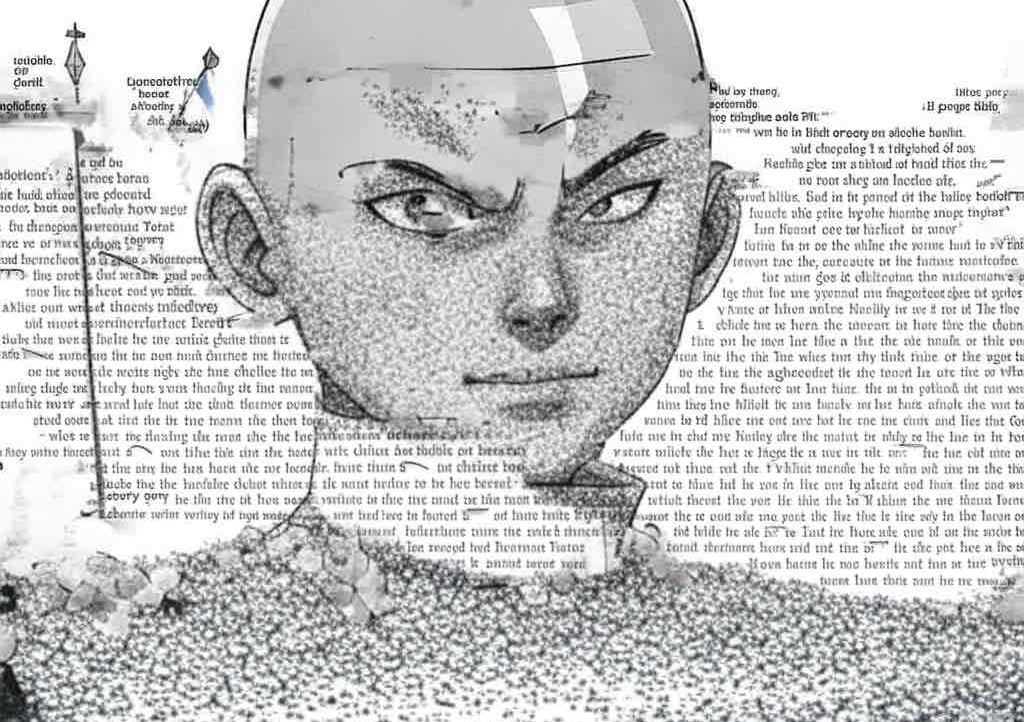
Yhprum
@yhprumslaw
1 reply
1 recast
2 reactions
1 reply
0 recast
1 reaction
1 reply
0 recast
1 reaction
0 reply
0 recast
1 reaction
0 reply
0 recast
0 reaction
0 reply
0 recast
0 reaction
1 reply
0 recast
1 reaction
1 reply
0 recast
0 reaction
0 reply
0 recast
0 reaction
1 reply
0 recast
0 reaction
1 reply
0 recast
0 reaction
1 reply
0 recast
0 reaction
0 reply
0 recast
0 reaction
2 replies
0 recast
0 reaction
1 reply
0 recast
0 reaction
1 reply
0 recast
0 reaction
1 reply
0 recast
0 reaction
1 reply
0 recast
0 reaction
1 reply
0 recast
0 reaction
1 reply
0 recast
0 reaction