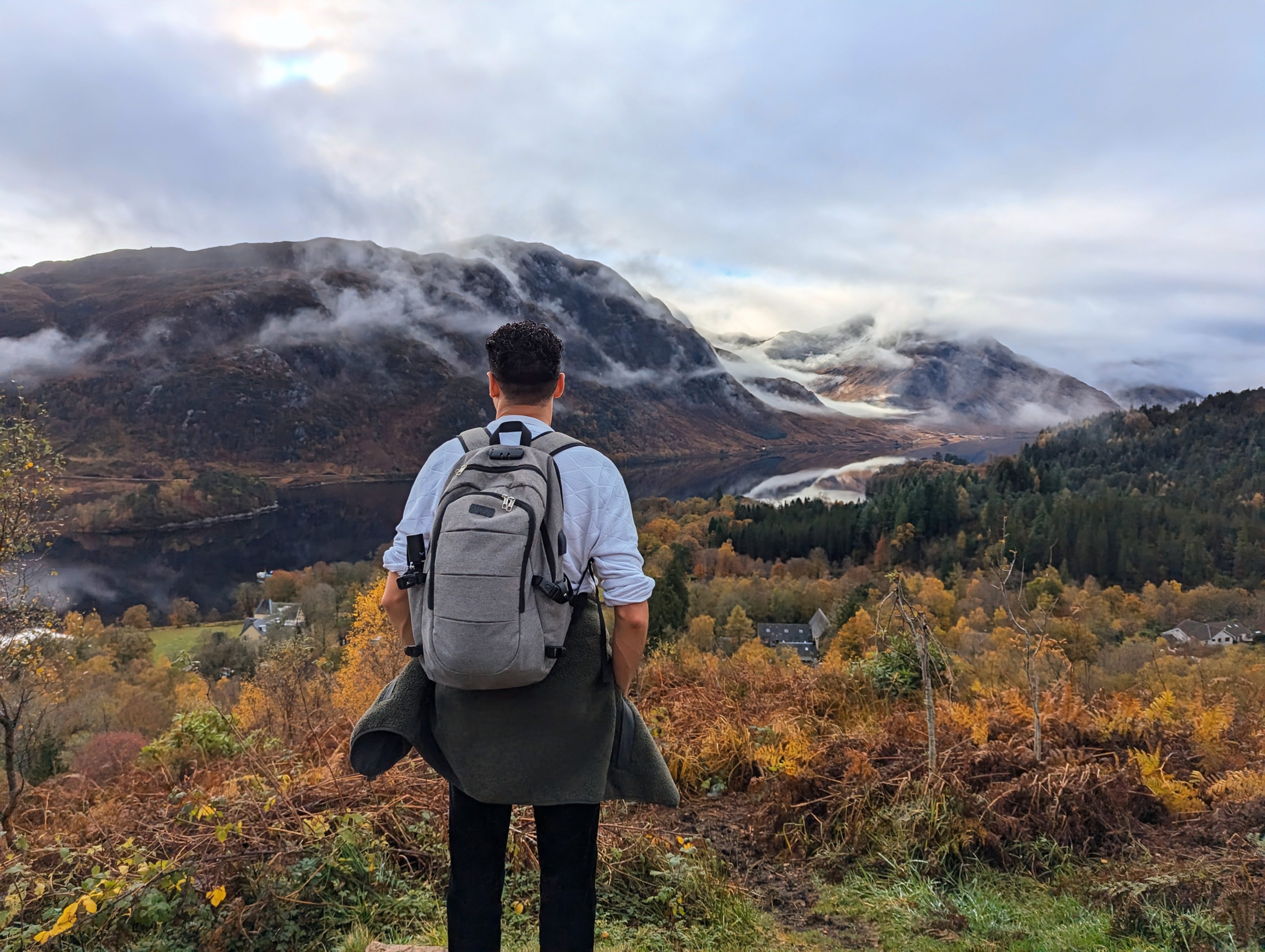
Makushi π§
@makushi
0 reply
0 recast
0 reaction
0 reply
0 recast
0 reaction
0 reply
0 recast
0 reaction
0 reply
0 recast
0 reaction
0 reply
0 recast
0 reaction
0 reply
0 recast
0 reaction
0 reply
3 recasts
2 reactions
0 reply
3 recasts
3 reactions
0 reply
2 recasts
3 reactions
0 reply
0 recast
1 reaction
0 reply
1 recast
1 reaction
0 reply
1 recast
1 reaction
0 reply
0 recast
1 reaction
0 reply
1 recast
1 reaction
0 reply
0 recast
0 reaction
0 reply
1 recast
1 reaction
0 reply
0 recast
0 reaction
0 reply
0 recast
0 reaction
0 reply
1 recast
1 reaction
0 reply
0 recast
0 reaction